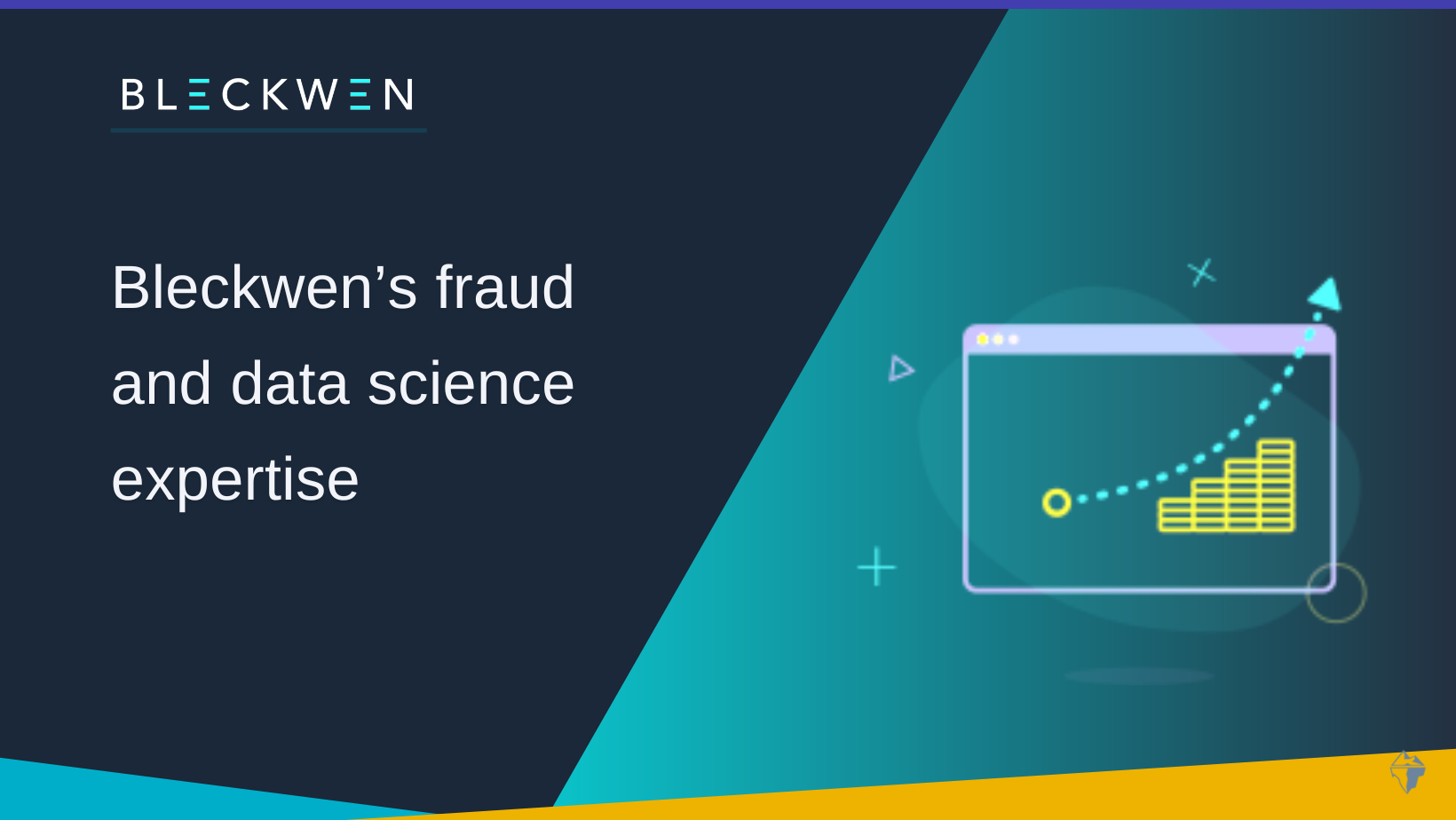
You know that you are losing money every day! You have started thinking about launching an AI-based anti-fraud program because you want to reduce residual fraud. Your initial thinking is that it should be easy enough with your data and a few data scientists.
However, you quickly realize that the scope and collaboration needed to deliver this project is immense - requiring not only your data science team but also time and resources from your risk, compliance, marketing, and IT teams. However, you quickly realize that the number of critical stakeholders needed is much more significant and collaboration much more comprehensive than you initially thought. In this post, I will describe the multidisciplinary team and timeline required for such a project.
The path to success for this kind of project involves the perfect alignment of stakeholders. As with any project, it begins with a kickoff meeting including the key stakeholders: Risk & Compliance (primary stakeholders), Marketing, IT, and your data science team (depending on your org, they are either attached to the business team or the IT team). You must ensure they understand the respective timelines, goals, and business constraints.
Next, you will hold a series of meetings to frame the project. Everyone in the company is busy with various constraints. Thus this phase of the project might end up lasting around 3 months. With half a dozen 2-hour meetings involving 3-5people, emails are exchanged. Of course, research on the topic is needed, too - just to evaluate the potential implications, estimate the ROI (return on investment), and finally, the required budget for the project.
After this 3-month framing period, you invest 30 M-days (Man days) to build a preliminary business case for budget approval. In parallel, fraudsters are stealing your money, yet you don’t have proof of potential savings.
Finally, you present the budget so you can launch the project. You’ve made assumptions and split the project into 3 phases:
As you have experience with IT projects, the historical data validation includes: Workshops, meetings, and committees with your data science, IT, risk, compliance, and marketing teams. Great, we’re moving! But all of them still need to learn the business complexity and constraints. In your mind, the main party involved in the project is your data science team. Can they do it in 3 months with just a part-time senior and full-time junior data scientist?
Are your data scientists experts in fraud detection models? Have you thought about the tools that they need? Do they need support from data engineers for the data preparation? By the end of this 3-month long validation period, you have invested 200 M-days, dealt with a few unexpected surprises, and finally have the model designed for offline analysis.
Let me do the math: you spent 230M days at 450€/day. Add in the cost of the extra resources needed: servers, licenses(5k€/month). You are playing poker and have just spent roughly 120 000 € just to stay in the game. After 6 months, you have finally reached a key milestone. You now know this will be a “go or no go”! Next is the building phase, where you need to industrialize the entire process.
There are two options: rely on a Data Science platform that you must accelerate. Or you can build your own technology. But you still need to consider how you will connect your data stream and the AI model lifecycle. Let’s assume that you decide to build on top of an existing solution. Your team will spend a couple of weeks on this model– building, training, and automation- and then it could take 6 months for the data integration. You invest 500-man days, including management committees, at the end of this period. All of which should align with the crazy company roadmap.
Have you thought about running/maintaining your models? IT teams are used to SLAs and software monitoring, but has anyone considered model monitoring yet? This is key as the model needs to be monitored to be efficient. This requires specific knowledge and expertise to understand the impact of feature drift and decide when the right time is to retrain the model. After 9 months, you are ready to go live. Crazy how time flies, isn’t it?
Let me do the math for you again. Not only did the fraudsters not stop during this period. But they actually improved, evolved, and changed their strategies. Now, you pushed a possibly accurate model 6 months ago. Your total investment represents 730M days. And don’t forget the cost of the extra resources (servers, licenses, etc.)? You have spent around 360,000€ and lost hundreds of thousands of euros to fraudsters.
Now that you are in the run phase of your project, your costs should be lower, but you still need to maintain the whole application. In addition to your usual IT costs, you need to consider the cost of model monitoring, model retraining, model updates, and data evolution - because fraudsters also adapt. Unfortunately, they adapt even faster than you. In this run phase, you need 25 M-days per month, add the necessary extra resources, and you’re at about 180 000€ per year.
Building and running your own AI fraud model by yourself may seem like a good option, but at the same time, it is a massive risk. Louis Colombus (principal at Dassault system) mentioned in the state of MLOps 2021 that an astounding 87% of ML projects never land in production. It’s critical to know that the cost of building your own model is not only comprised of the initial investment.
But also, running it costs much higher than standard apps. Let alone that, in this specific fraud context, you’re losing money every day while your system is not in place. It is a tradeoff between ownership, long-term strategy, immediate ROI, and efficiency.
Proven results & guaranteed fraud savings
Tailored for your business
Easy to integrate with rapid time to value